In industries ranging from manufacturing to aviation, RUL helps organizations optimize maintenance schedules, reduce downtime, and maximize operational efficiency. By predicting when equipment might fail, businesses can avoid costly breakdowns and ensure smooth operations. Understanding RUL not only enhances decision-making but also supports sustainability by prolonging the life of assets. This guide will delve into the intricacies of RUL, exploring its applications, methodologies, and benefits. The concept of RUL has gained prominence with advancements in technology, particularly in artificial intelligence, machine learning, and the Internet of Things (IoT). These innovations enable real-time data collection and analysis, making RUL predictions more accurate and actionable than ever before. Industries such as healthcare, energy, and transportation have embraced RUL to improve safety, reduce costs, and enhance performance. For instance, in aviation, RUL predictions help airlines schedule engine maintenance before a potential failure, ensuring passenger safety and minimizing delays. Similarly, in manufacturing, RUL allows companies to anticipate equipment wear and tear, avoiding costly production halts. In this article, we will explore the multifaceted aspects of RUL, answering key questions such as "How is RUL calculated?" and "What are the benefits of implementing RUL in predictive maintenance?" We'll also discuss the challenges associated with RUL estimation and provide practical insights into its applications across various industries. By the end of this guide, you'll have a comprehensive understanding of RUL and how it can transform your approach to asset management and maintenance.
Table of Contents
- What is RUL, and Why Does It Matter?
- How is RUL Calculated?
- What Are the Benefits of RUL in Predictive Maintenance?
- Applications of RUL Across Industries
- Challenges in Estimating RUL
- How Can Organizations Implement RUL Effectively?
- Tools and Technologies for RUL Prediction
- Frequently Asked Questions About RUL
What is RUL, and Why Does It Matter?
RUL, or Remaining Useful Life, is a metric used to estimate the time left before a system or component reaches the end of its operational life. This concept is particularly important in industries where equipment failure can lead to significant financial losses, safety risks, or operational disruptions. By understanding RUL, organizations can make informed decisions about maintenance schedules, resource allocation, and asset replacement.
The importance of RUL extends beyond just preventing failures. It plays a pivotal role in optimizing operational efficiency and reducing costs. For example, in the manufacturing sector, knowing the RUL of machinery allows companies to schedule maintenance during non-peak hours, minimizing the impact on production. Similarly, in the energy sector, RUL helps utilities predict when critical infrastructure, such as wind turbines or power transformers, might fail, enabling proactive measures to prevent outages.
Read also:Alan Osmonds Wealth Revealed Insights Into His Life And Legacy
Moreover, RUL contributes to sustainability efforts by extending the lifespan of assets. Instead of prematurely replacing equipment, organizations can use RUL predictions to maximize the utility of existing resources. This not only reduces waste but also aligns with global efforts to promote environmentally responsible practices. In short, RUL is a cornerstone of modern asset management, offering a data-driven approach to maintaining operational excellence.
How is RUL Calculated?
Calculating RUL involves a combination of data analysis, statistical modeling, and machine learning techniques. The process begins with the collection of operational data from sensors, historical records, and other sources. This data is then analyzed to identify patterns and trends that indicate the health and performance of the asset.
What Are the Common Methods for Estimating RUL?
There are several methodologies for estimating RUL, each with its own strengths and limitations. Below are some of the most widely used approaches:
- Physics-Based Models: These models rely on the physical properties and behavior of the asset. They use mathematical equations to simulate how a component degrades over time.
- Data-Driven Models: These models leverage historical data and machine learning algorithms to predict RUL. Techniques such as regression analysis, neural networks, and decision trees are commonly employed.
- Hybrid Models: Combining physics-based and data-driven approaches, hybrid models offer a more comprehensive and accurate estimation of RUL.
What Role Does Machine Learning Play in RUL Estimation?
Machine learning has revolutionized RUL estimation by enabling the analysis of large and complex datasets. Algorithms such as support vector machines (SVM), random forests, and deep learning models can identify subtle patterns in data that traditional methods might miss. For instance, in aviation, machine learning models analyze sensor data from aircraft engines to predict when maintenance is required.
One of the key advantages of machine learning is its ability to adapt and improve over time. As more data becomes available, the models can refine their predictions, leading to greater accuracy. This adaptability makes machine learning an invaluable tool for RUL estimation, particularly in dynamic environments where conditions are constantly changing.
What Are the Benefits of RUL in Predictive Maintenance?
RUL is a cornerstone of predictive maintenance, offering numerous advantages that enhance operational efficiency and reduce costs. By predicting when equipment is likely to fail, organizations can avoid unexpected breakdowns and schedule maintenance activities more effectively.
Read also:Adorable World Of Sanrio A Guide To Hello Kitty Characters
How Does RUL Enhance Predictive Maintenance Strategies?
RUL enables predictive maintenance by providing actionable insights into the health of assets. Instead of relying on fixed maintenance schedules, which may result in unnecessary interventions, organizations can use RUL predictions to perform maintenance only when needed. This not only saves time and resources but also minimizes the risk of over-maintenance, which can lead to premature wear and tear.
Additionally, RUL helps prioritize maintenance tasks based on urgency. For example, if the RUL of a critical piece of equipment is short, it can be addressed immediately, while less critical assets with longer RULs can be scheduled for later. This prioritization ensures that resources are allocated efficiently, maximizing the impact of maintenance efforts.
What Are the Cost Implications of Using RUL in Maintenance?
Implementing RUL in predictive maintenance can lead to significant cost savings. By reducing unplanned downtime, organizations can avoid the financial losses associated with halted production, missed deadlines, and emergency repairs. Moreover, extending the lifespan of assets through timely maintenance reduces the need for costly replacements.
For instance, in the automotive industry, RUL predictions help manufacturers identify potential issues in vehicles before they become critical. This proactive approach not only enhances customer satisfaction but also reduces warranty claims and repair costs. Similarly, in the energy sector, RUL helps utilities optimize the maintenance of power plants, ensuring reliable electricity supply while minimizing expenses.
Applications of RUL Across Industries
RUL is not limited to a single industry; its applications span a wide range of sectors, each benefiting from its predictive capabilities. From healthcare to transportation, RUL is transforming how organizations manage their assets and operations.
How is RUL Used in the Aviation Industry?
In aviation, RUL plays a crucial role in ensuring the safety and reliability of aircraft. By predicting the remaining life of critical components such as engines, landing gear, and avionics, airlines can schedule maintenance activities to prevent failures. This proactive approach not only enhances passenger safety but also reduces operational disruptions and costs.
For example, airlines use RUL predictions to determine when engine overhauls are necessary. By performing maintenance before a potential failure, they can avoid costly delays and cancellations. Additionally, RUL helps airlines comply with regulatory requirements, ensuring that all equipment meets safety standards.
What Are the Benefits of RUL in the Manufacturing Sector?
In manufacturing, RUL is used to optimize production processes and reduce downtime. By predicting when machinery is likely to fail, manufacturers can schedule maintenance during non-peak hours, minimizing the impact on production. This not only improves efficiency but also enhances product quality by ensuring that equipment operates at optimal performance levels.
Moreover, RUL helps manufacturers extend the lifespan of their assets. Instead of replacing equipment prematurely, they can use RUL predictions to maximize the utility of existing resources. This approach not only reduces costs but also supports sustainability efforts by minimizing waste.
Challenges in Estimating RUL
While RUL offers numerous benefits, estimating it accurately can be challenging. Several factors, such as data quality, model complexity, and environmental conditions, can impact the accuracy of RUL predictions.
What Are the Common Challenges in RUL Estimation?
One of the primary challenges in RUL estimation is the availability of high-quality data. Accurate predictions require comprehensive and reliable data, which may not always be available. In some cases, sensors may malfunction, or historical records may be incomplete, leading to inaccurate models.
Another challenge is the complexity of the models used for RUL estimation. While advanced techniques such as machine learning offer greater accuracy, they also require significant computational resources and expertise. Organizations may struggle to implement these models without the necessary infrastructure and skilled personnel.
How Can Environmental Factors Affect RUL Predictions?
Environmental conditions, such as temperature, humidity, and vibration, can significantly impact the performance and lifespan of assets. For example, equipment operating in harsh environments may degrade faster than expected, leading to shorter RULs. Accounting for these variables in RUL models can be challenging, particularly in dynamic settings where conditions are constantly changing.
To address these challenges, organizations must invest in robust data collection systems, advanced analytics tools, and skilled personnel. By doing so, they can improve the accuracy of RUL predictions and maximize the benefits of predictive maintenance.
How Can Organizations Implement RUL Effectively?
Implementing RUL effectively requires a strategic approach that combines technology, processes, and people. Organizations must ensure that they have the necessary infrastructure, expertise, and resources to leverage RUL for predictive maintenance and asset management.
What Steps Should Organizations Take to Adopt RUL?
The first step in implementing RUL is to establish a robust data collection system. This involves installing sensors on critical assets to monitor their performance in real-time. The data collected should be comprehensive, accurate, and reliable to ensure the effectiveness of RUL predictions.
Next, organizations should invest in advanced analytics tools and machine learning models to analyze the data and estimate RUL. These tools should be tailored to the specific needs and challenges of the organization, ensuring that they provide actionable insights. Additionally, organizations should train their personnel to use these tools effectively, enabling them to interpret the results and make informed decisions.
How Can Organizations Overcome Implementation Challenges?
One of the key challenges in implementing RUL is resistance to change. Employees may be hesitant to adopt new technologies or processes, particularly if they perceive them as disruptive. To overcome this, organizations should communicate the benefits of RUL clearly and involve employees in the implementation process.
Another challenge is the cost of implementation. While RUL can lead to significant cost savings in the long term, the initial investment in technology and training can be substantial. To address this, organizations should develop a phased implementation plan, starting with pilot projects to demonstrate the value of RUL before scaling up.
Tools and Technologies for RUL Prediction
Several tools and technologies are available to support RUL prediction, ranging from software platforms to hardware solutions. These tools enable organizations to collect, analyze, and interpret data effectively, enhancing the accuracy of RUL predictions.
What Are the Key Features of RUL Prediction Tools?
RUL prediction tools typically include features such as data visualization, real-time monitoring, and predictive analytics. These features enable organizations to track the health of their assets, identify potential issues, and make informed decisions about maintenance and replacement.
For example, platforms such as IBM Maximo and SAP Predictive Maintenance use machine learning algorithms to analyze sensor data and predict RUL. These platforms also offer dashboards and reports that provide a clear overview of asset performance, helping organizations prioritize maintenance tasks.
How Can IoT Enhance RUL Prediction?
The Internet of Things (IoT) plays a crucial role in RUL prediction by enabling real-time data collection and analysis. IoT devices, such as sensors and smart meters, provide continuous monitoring of asset performance, allowing organizations to detect issues early and
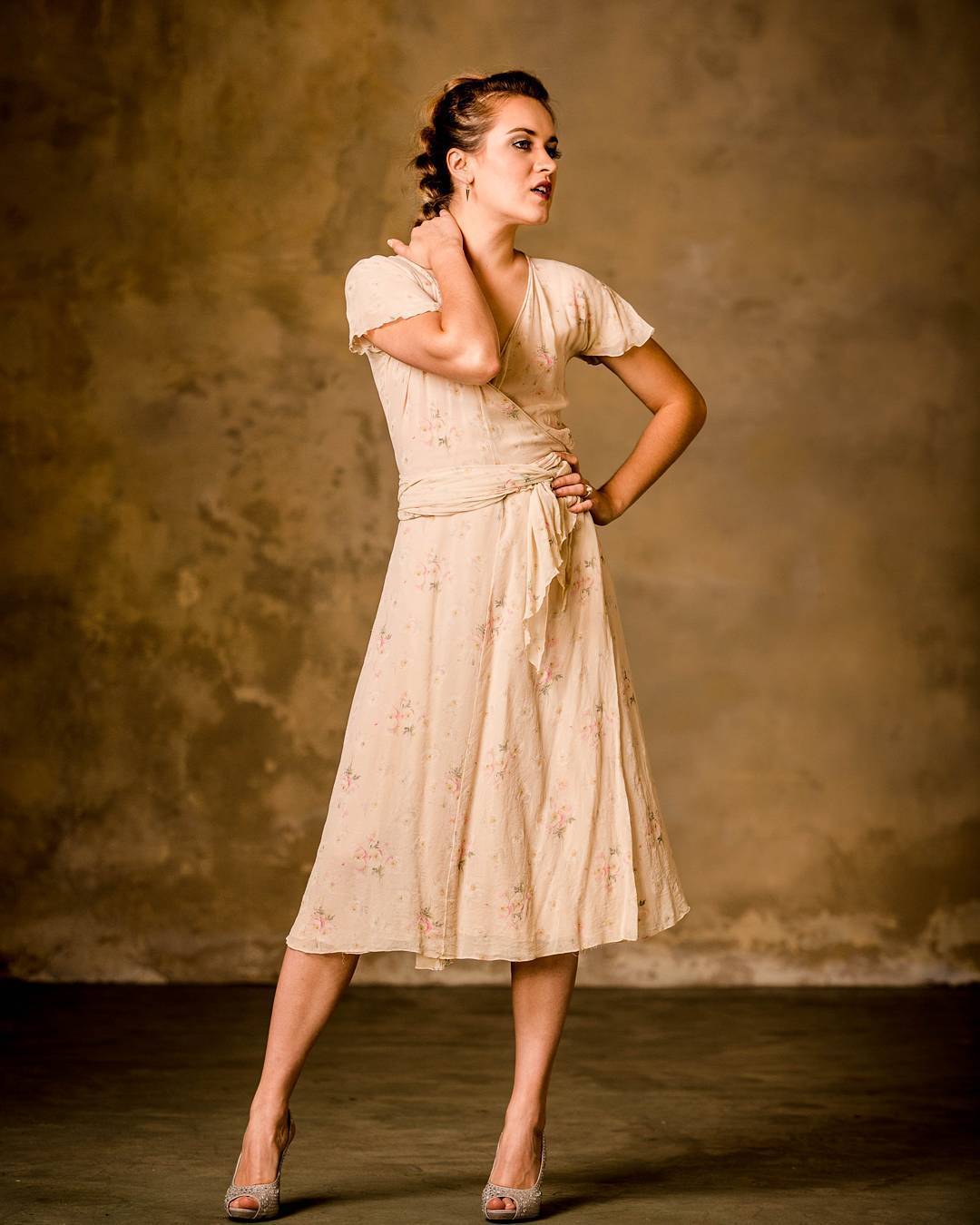
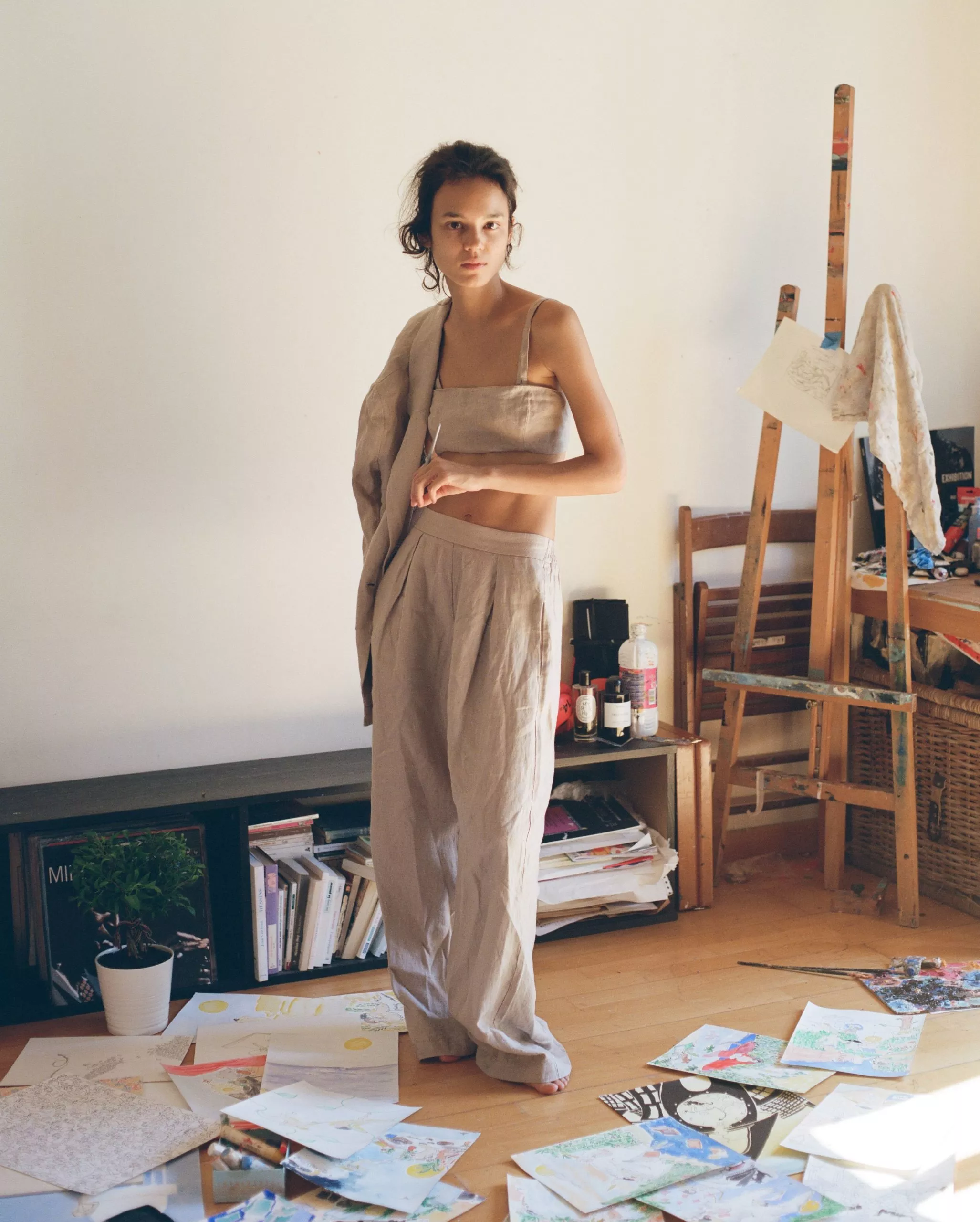